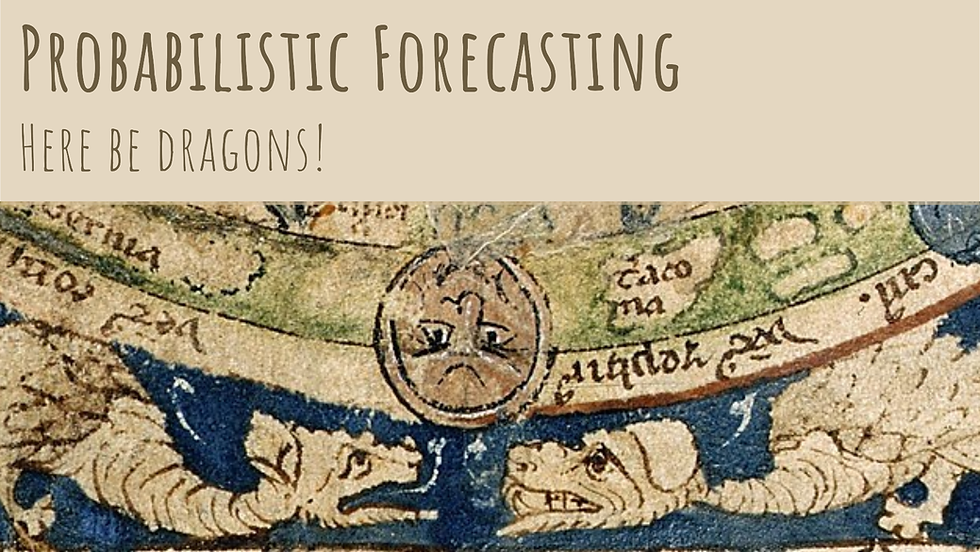
If you look at pictures of medieval maps, you'll notice on some of them prominent signs reading "Here be dragons!" to warn travellers of uncharted areas believed to host dangers of various kinds (like monstrous creatures or unexplained phenomena).
We've all been faced with answering the question of "how long is this going to take?", no matter how much we try to push against it. In recent times, the idea of answering this question using some form of probabilistic technique has received a lot of traction, and it's definitely a step forward compared to other approaches we used in the past that relied on practices that, very often, weren't much more sophisticated that crystal ball gazing. But at the same time, there are some considerations we need to keep in mind to make sure we're not taking unnecessary risks. In other words: "Here be dragons!"
You can always do the math, but...
Probabilistic forecasting can take different forms, from looking at Lead Time distributions to using Monte Carlo simulations. Something that I think it's important to remember is that you can always use a formula, consult a look-up table, or enter parameters in a simulator and run it many times. But the fact that you can do math doesn't mean that the results are trustworthy.
Here are some questions to consider to establish how much you can trust your math, as well as some "TL;DR" commentary for them (let me know in the comments which one you'd like to see expanded in a separate article 😉)
What kind of volatility are you seeing in your process?
TL;DR: Look at data coming out of your system in some form of run chart. Are you seeing "stable volatility"? You're using the past to predict the future, so you need to have good reasons to believe the future will look like the past. If instead you see the system "trending" (moving "somewhere else", because, for example, you're introducing new techniques, practices, or organizational changes) then a forecast based on the past can be very unreliable.
What do you know about the distribution of single items?
TL;DR: Are you seeing a normal (symmetrical, "Gaussian") distribution? or is it skewed (a "hump" to one side and a long "tail" towards the other)? How skewed is it? (exponential, sub-exponential, super-exponential) This will tell you things like how representative averages are, how big your samples need (or can) be, how quickly your system converges to the average, and more in general, how unpredictable it can be.
If you're seeing lots of variability in single items (very "extreme" distributions), then forecasting of batches is unadvisable (or even mathematically unsound). You need to work on stabilizing the delivery of single items first ("trimming the tail", and also likely "left shifting" the distribution). Practices like Service Delivery Reviews (SDRs) and Blocker Clustering are your friends here.
If you're about to use a sampling simulator (like a Monte Carlo simulator working from a sample), how representative is the sample you're about to use?
TL;DR: The key here is that the sample needs to be random. Keep in mind that "the last N items we delivered" is not a random sample of your process. The sample also needs to be "big enough"; in many cases, this means a sample so big that it's impractical to collect. If the system is also "trending" (see the first point above), by the time you collect all the needed samples the system will be somewhere else and the sample, while "big enough", might not be representative.
If you're about to use a parametric simulator (like a Monte Carlo simulator working from formulas with parameters, rather than an "empirical sample"), how well does the model match your actual process, and how much confidence do you have in the parameters you're setting?
TL;DR: you need to understand how your work works to evaluate if the simulator matches your process, and how to configure it. In particular, what kinds of sources of delay are you most exposed to, what are their probabilities of occurring, and their impact. Once more, feedback mechanisms like the SDR can help you gain these insights over time.
What do you know about "dark matter" expansion in your context?
TL;DR: As it turns out, when delivering a batch of items together (as it happens in a project, or when building a new product, for example), the biggest uncertainty actually comes from determining the total number of items in the batch. It's not uncommon to find that new work is discovered as we complete other work; this is work that "was always there" but we weren't able to initially see (because the delivery process is in itself a knowledge generation process). Practices that help you mitigate "dark matter expansion" (as well as understand to what degree your process tends to generate it) include the use of Customer Recognizable work, deliberate Upstream process activities, and feedback loops (such as the SDR).
"OK, is it then back to crystal ball gazing?"
Not at all. The list of questions above are not meant to deter you from using things like Monte Carlo simulations and other forms of probabilistic thinking. On the contrary, it's meant to encourage its use in a way that it's appropriate and informed.
In the past, we've collectively fallen for simplistic estimation methods (like counting story points and drawing burn-down charts). Now we know better, but we need to understand the nuance and have realistic expectations, otherwise we risk the naive use of much more sophisticated tools, with all the risk that includes.
Here's an example of what I mean: from reading the items above, you might conclude that you need to wait until you have lots of data points, or an accurate, very refined model of your process before you can start using probabilistic techniques. The advice here is not to wait that long. Use the data you have now, but understand the limits of the results you're seeing, use multiple models to contrast answers, and above all, start working on understanding how your work really works, and use those insights to introduce improvements NOW.
The dragons are, of course, in the details: there's a great deal of nuance behind each of the points raised here, which I'm planning to address in subsequent articles. Let me know in the comments which one feels more relevant to you today, and I'll start from there.
To close with another Alexism: "An improved service is better than a more precise model of an unsatisfactory service". Take action to improve today.
Thanks to my colleague and friend Alexei Zheglov, from whom I learnt all of these concepts, and helped me walk away from "crystal ball gazing" and "tea leaf reading" 😉
Commentaires